Helppoa kuin mallinteko – lyhyt oppimäärä elinaikamallinnukseen ja ekstrapoloinnin aiheuttamaan epävarmuuteen taloudellisessa arvioinnissa
Keywords:
ekstrapolointi, eloonjäämisanalyysi, epävarmuus, terveystaloudellinen arviointiAbstract
It’s not rocket science – or is it? A short introduction to survival modelling and uncertainties in extrapolation in economic evaluation
To support decision-making on the reimbursement or implementation of new pharmaceuticals, the additional value and costs of new treatments are appraised through health economic modelling. Research data related to the effects of new treatments are often from too short period compared to the need; thus, the effects must be extrapolated for a longer period. This disparity in timespans increases the uncertainty associated with modelling results and decision-making. This study introduces simple survival modelling and demonstrates the effects of model selection and the duration of the follow-up time available on the accuracy of a survival model of an immuno-oncological therapy.
The material used was open-label synthetic data based on follow-up data from a clinical trial surveying a treatment of myeloid leukaemia. The effect of the follow-up duration on the success of the survival model was examined by cutting the follow-up data at one, two, and three years and then modelling the cut-off data using commonly employed parametric distributions. The resulting survival models were compared to the survival curves of the entire data set, and accuracy was assessed based on survival rate and restricted mean survival time at three- and six-year time points. The most successful models were predicted using cumulative risk curves and information criteria.
All models underestimated survival based on survival rates and restricted mean survival time compared to the entire data set. There were considerable differences in outcomes between the models. Longer follow-up time improved the accuracy. The risk curves and information criteria were not able to predict the most accurate model.
The applied survival model and length of the follow-up data significantly affect the accuracy of the model when modelling health outcomes of new pharmaceuticals. The specific characteristics of immuno-oncological therapies, such as rapid risk change and long-term survival, must be considered when selecting the model and assessing uncertainty. There are tools available to support the choice of model and assessment of the result, but they can be used only to compare the model to the follow-up data. The flexible modelling techniques may add to the preciseness of the model. To succeed in survival modelling, researchers must perceive and assess the properties of the employed data, the choice of the survival model, the credibility of the extrapolation and the sources of uncertainty. The uncertainty about the result of an extrapolated survival model cannot be eliminated from the model completely.
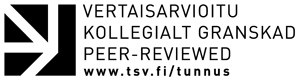